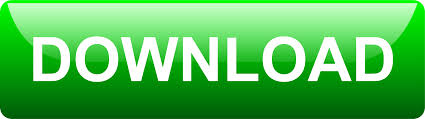
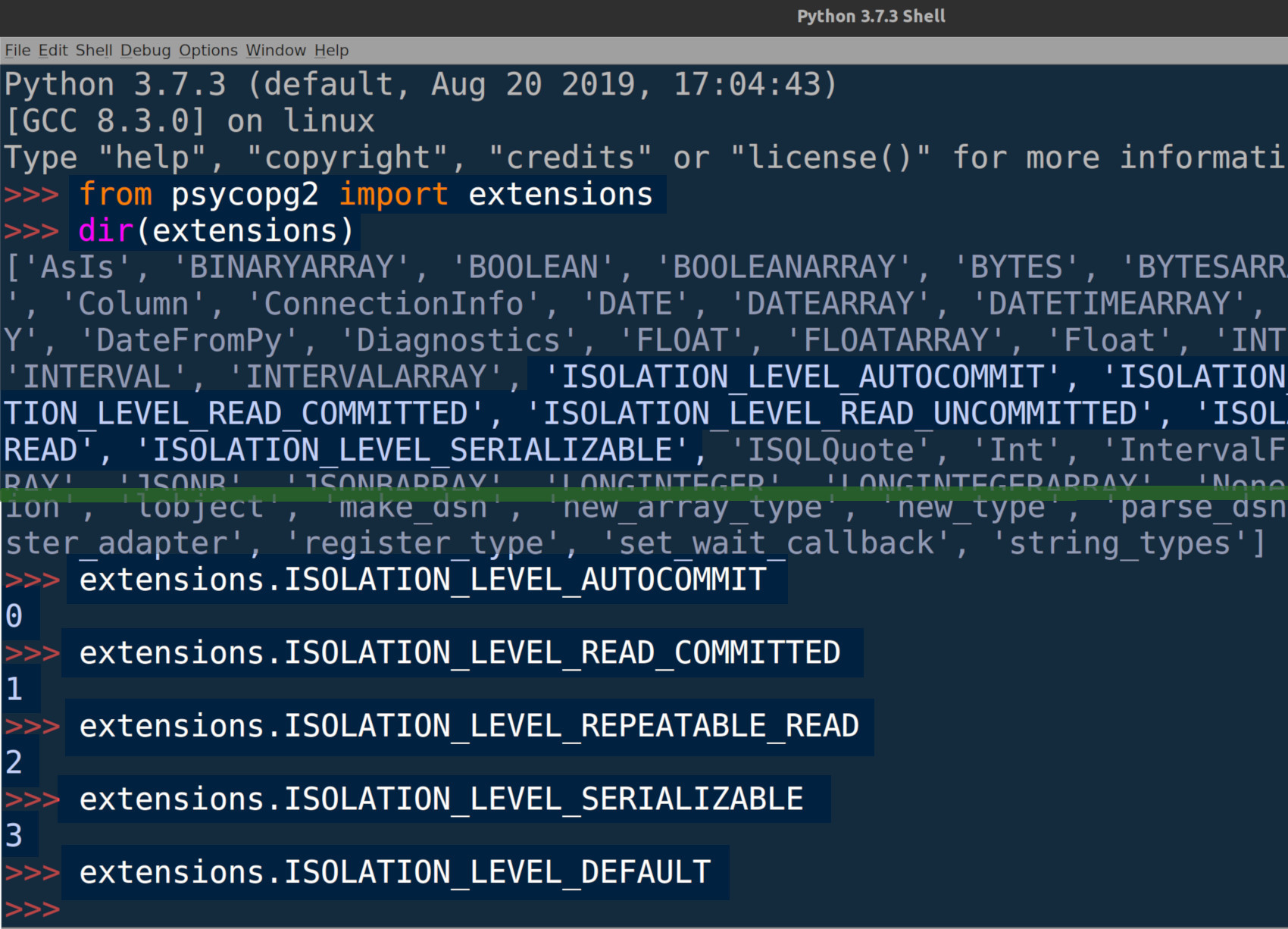
But once I tried using a database to perform my data munging tasks - specifically PostgreSQL and TimescaleDB - I realized how efficient and straightforward analysis, and particularly cleaning tasks, could be when done directly in a database.īefore using a database for data cleaning tasks, I would often find either columns or values that needed to be edited. Throughout my time in this job, I got the chance to use many popular data analysis tools including Excel, R, and Python. My role at this company was to perform data analysis and business intelligence tasks. Cleaning may not be the most glamorous step in the analysis process, but it is absolutely crucial to creating accurate and meaningful models.Īs I mentioned in my last post, my first job out of college was at an energy and sustainability solutions company that focused on monitoring all different kinds of utility usage - such as electricity, water, sewage, you name it - to figure out how our clients’ buildings could be more efficient. I now want to move on to the second step, data cleaning. In my previous post, I focused on showing data evaluation techniques and how you can replace tasks previously done in Python with PostgreSQL and TimescaleDB code. However, once I was introduced to PostgreSQL and TimescaleDB, I found how efficient and fast it was to do my data munging directly within my database. Historically, I have done my data munging and modeling all within Python or R, these being excellent options for analysis. The first three steps of the analysis lifecycle (evaluate, clean, transform) comprise the “data munging” stages of analysis.

Python postgresql series#
I began this series of posts on data analysis by presenting the following summary of the analysis process: Data Analysis Lifecycle TimescaleDB and PostgreSQL cannot replace these tools entirely, but they can help your data munging/cleaning tasks be more efficient and, in turn, let Excel, R, and Python shine where they do best: in visualizations, modeling, and machine learning.Ĭleaning is a very important part of the analysis process and generally can be the most grueling from my experience! By cleaning data directly within my database, I am able to perform a lot of my cleaning tasks one time rather than repetitively within a script, saving me considerable time in the long run. In this blog post, I explore some classic data cleaning scenarios and show how you can perform them directly within your database using TimescaleDB and PostgreSQL, replacing the tasks that you may have done in Excel, R, or Python. Sometimes to properly evaluate your data, you may need to do some pre-cleaning before you get to the main data cleaning, and that’s a lot of cleaning! In order to accomplish all this work, you may use Excel, R, or Python, but are these the best tools for data cleaning tasks? During analysis, you rarely-if ever-get to go directly from evaluating data to transforming and analyzing it.
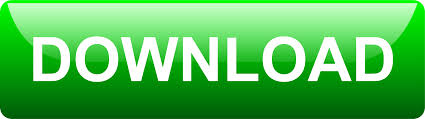